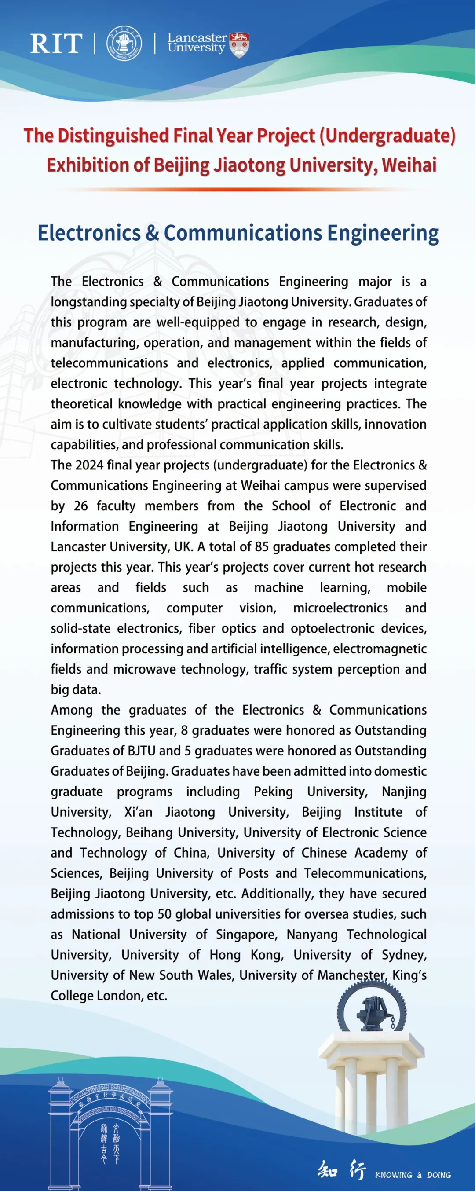
Zheng Xiaoshan
This study is based on the Raspberry Pi 4B and utilizes a multi-sensor system to monitor the pulse wave of the radial artery. By collecting and analyzing pulse signals, including pulse rate, pulse wave velocity, and pulse classification, it enables diagnostic and therapeutic support for cardiovascular health and potential pathological changes. Wavelet decomposition, empirical modal decomposition, and time-frequency domain analysis methods, combined with correction and preprocessing techniques, were used to obtain multidimensional pulse wave data.
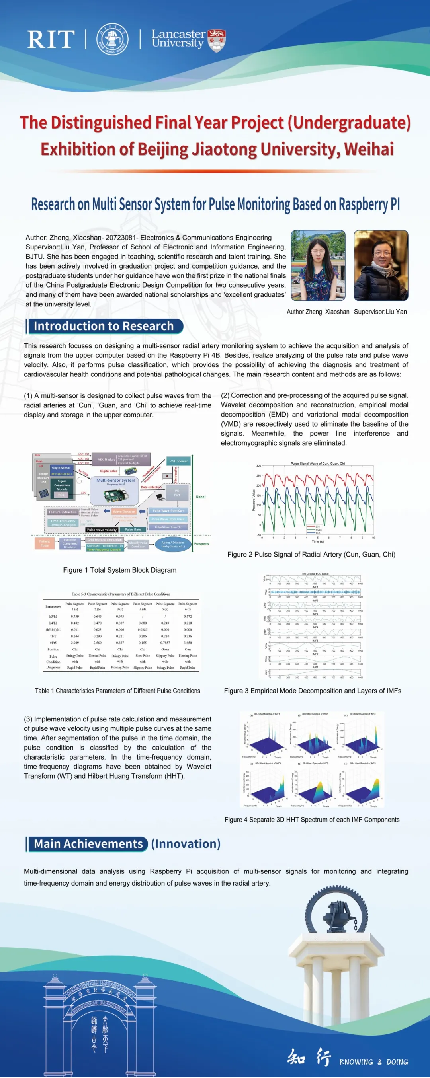
Liao Jianhua
This study is dedicated to the development of a neurofeedback system based on low-cost EEG signal acquisition circuitry, which provides high-quality EEG signals with adjustable DC bias and amplification, and realizes the amplification of Alpha wave and the removal of IF interference through an Arduino board, and uses Jupyter notebook for the feature scaling and FFT filtering of the data, while Brainbay software offers The Brainbay software provides real-time processing and acoustic/visual feedback functions, and realizes electronic filtering, amplitude adjustment, and specific frequency extraction of EEG signals through a modular interface and low-code scheme.
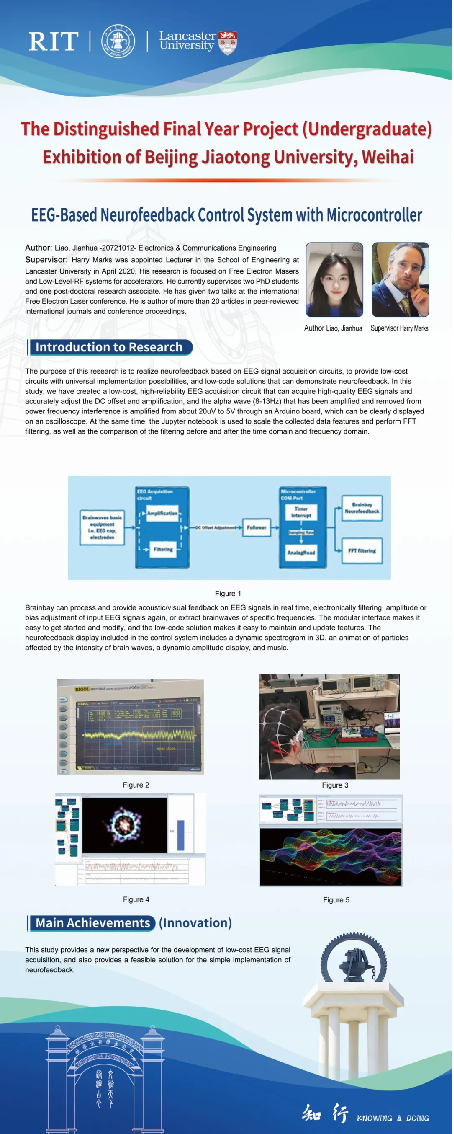
He Xudong
This study proposes a regional traffic situational awareness and assessment system based on a UAV vision platform, focusing on improving the YOLOv8 algorithm to enhance the detection of overhead vehicle targets, introducing the SimAM attention mechanism and enhancing the small target detection head. In addition, flexible applications in complex urban environments are realized by transforming remote sensing image coordinates through trigonometric operations and dynamic route planning based on ant colony algorithm.
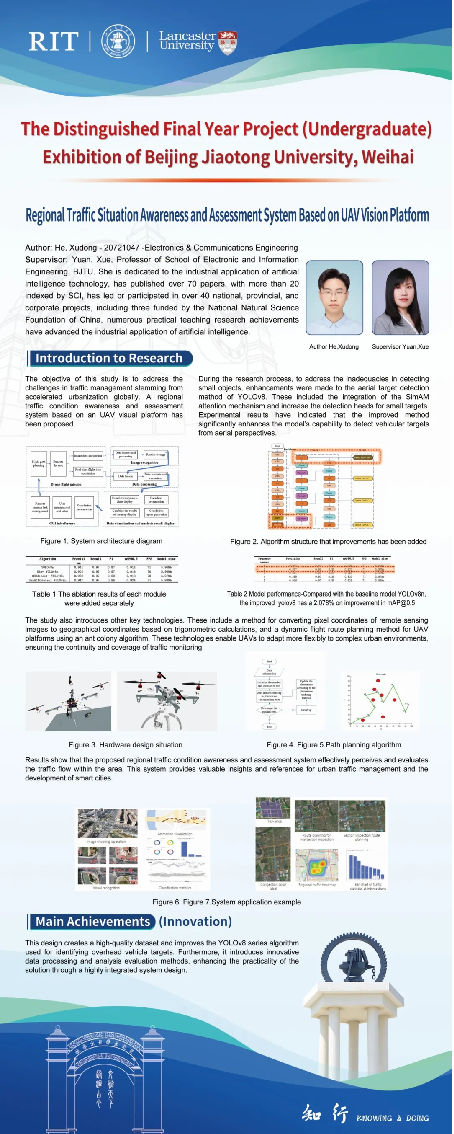
Luo Binwei
In this project, a lightweight epilepsy prediction algorithm is investigated, which significantly reduces the algorithm complexity and network parameters through channel selection and optimization of the neural network structure to adapt to the needs of portable and wearable devices. The algorithm uses CNN-LSTM simplified EEGNet-GRU network for binary classification, and the prediction accuracy is evaluated by sample and event benchmarks, with an average precision of 78%, a recall of 84%, and an F1-score of 0.81; in the event evaluation, the average prediction rate is 92% and the average false alarm rate is 9%.
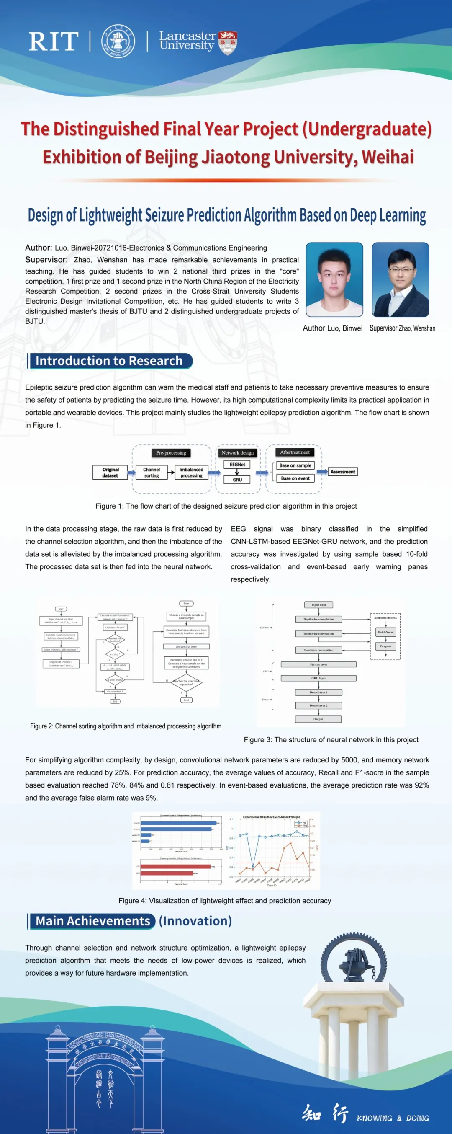
Wen Yueli
In this paper, a secure distributed power trading platform is constructed based on Hyperledger Fabric, which breaks through the limitations of traditional centralized management. The introduction of private datasets ensures the isolation of node information, while the combination of a partially centralized government regulatory mechanism improves transaction security and transparency. Real-time information query and performance analysis through CouchDB verified the integrity and reliability of the transaction process.
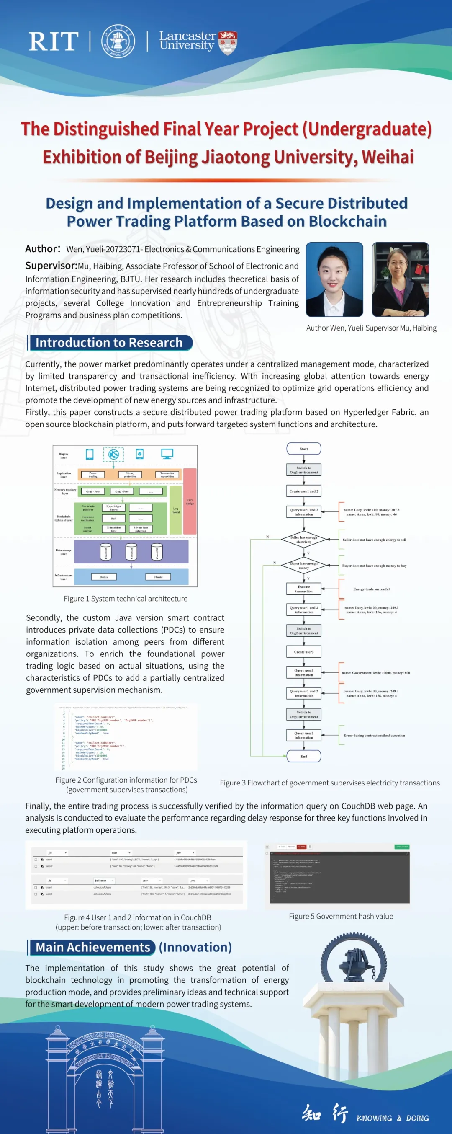